- By John Funk
- May 20, 2024
- ISA
- Feature
Summary
Machine learning is sometimes considered a subset of overarching AI. But in the context of digital security, it may be better understood as a driving force, the fuel powering the engine.
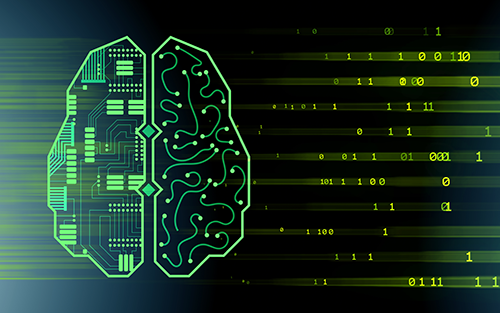
The terms “AI” and “machine learning” are often used interchangeably by professionals outside the technology, managed IT and cybersecurity trades. But, truth be told, they are separate and distinct tools that can be coupled to power digital defense systems and frustrate hackers.
Artificial iIntelligence has emerged as an almost ubiquitous part of modern life. We experience its presence in everyday household robots and the familiar Alexa voice that always seems to be listening. Practical uses of AI mimic and take human behavior one step further. In cybersecurity, it can deliver 24/7 monitoring, eliminating the need for a weary flesh-and-blood guardian to stand a post.
Machine learning is sometimes considered a subset of overarching AI. But in the context of digital security, it may be better understood as a driving force, the fuel powering the engine. Using programmable algorithms, it recognizes sometimes subtle patterns. This proves useful when deployed to follow the way employees and other legitimate network users navigate systems. Although even discussions regarding AI and machine learning feel redundant, to some degree, they are a powerful one-two punch in terms of automating security decisions.
The relationship between AI and machine learning in cybersecurity
Integrating AI calls for a comprehensive understanding of mathematics, logical reasoning, cognitive sciences and a working knowledge of business networks. The professionals who implement AI for security purposes must also possess high-level expertise and protection planning skills. Used as a problem-solving tool, AI can provide real-time alerts and take pre-programmed actions. But it cannot effectively stem the tide of bad actors without support. Enter machine learning.
In this context, machine learning emphasizes software solutions driven by data analysis. Unlike human information processing limitations, machine learning can handle massive swaths of data. What machine learning “learns,” for lack of a better word, translates into actionable security intel for the overarching AI umbrella.
Some people think about machine learning as a subcategory of AI, which it is. Others comprehend it in a functional way, i.e., two sides to the same coin. But for cybersecurity experts determined to deter, detect and repel threat actors, machine learning is the gasoline that powers AI engines.
How AI and machine learning are integrated into business networks
It’s now essential to leverage machine learning capabilities to develop a so-called “intelligent” computer that can defend itself, to some degree. Although the relationship between AI and machine learning is diverse and complex, an expert can integrate them into a cybersecurity posture with relative ease. It’s simply a matter of repetition and the following steps.
- Step 1: Create an AI system that relies on machine learning techniques.
- Step 2: Professionals then optimize machine learning by following data and use patterns.
- Step 3: Machine learning studies the patterns.
- Step 4: Repeat the process until the AI machine learning results achieve the needed accuracy level.
When properly orchestrated and refined to detect user patterns and subtle anomalies, the AI-machine learning relationship helps cybersecurity professionals keep valuable and sensitive digital assets away from prying eyes and greedy digital hands.
What are the benefits of coupling AI and machine learning?
First and foremost, it’s crucial to put AI and machine learning benefits in context. Studies consistently conclude that more than 80% of all cybersecurity failures are caused by human error. Using automated technologies removes many mistake-prone employees and other network users from the equation. Along with minimizing risk, these are benefits of onboarding these automated next-generation technologies.
Improved cybersecurity efficiency. According to the 2023 Global Security Operations Center Study, cybersecurity professionals spend one-third of their workday chasing down false positives. This waste of time negatively impacts their ability to respond to legitimate threats, leaving a business at higher than necessary risk. The strategic application of AI and machine learning can be deployed to recognize harmless anomalies and alert a CISO or vCISO only when authentic threats are present.
Increased threat hunting capabilities. Without proactive, automated security measures like MDR (managed detection and response), organizations are too often following an outdated break-and-fix model. Hackers breach systems or deposit malware, and then the IT department spends the remainder of their day, or week, trying to purge the threat and repair the damage. Cybersecurity experts have widely adopted the philosophy that “the best defense is a good offense.” A thoughtful AI-machine learning strategy can engage in threat hunting without ever needing a coffee break.
Cure business network vulnerabilities. Vulnerability management approaches generally employ technologies that provide proactive automation. They close cybersecurity gaps and cure inherent vulnerabilities by identifying these weaknesses and alerting human decision-makers. Unlike scheduling a routine annual risk assessment, these cutting-edge technologies deliver ongoing analytics and constant vigilance.
Resolve cybersecurity skills gap. It’s something of an open secret that there are not enough trained, certified cybersecurity experts to fill corporate positions. That’s one of the reasons why industry leaders tend to outsource managed IT and cybersecurity to third-party firms. Outsourcing helps to onboard the high-level knowledge and skills required to protect valuable digital assets and sensitive information. Without enough cybersecurity experts to safeguard businesses, automation allows the resources available to companies to drill down and identify true threats. Without these advanced technologies being used to bolster network security, it’s likely the number of debilitating cyberattacks would grow exponentially.
Industries that can benefit from automated AI and machine learning security
The type of predictive analytics and swift decision-making capabilities this two-prong approach delivers has seemingly endless industry applications. Banking and financial sector organizations can not only use AI and machine learning to repel hackers but also ferret out fraud. Healthcare organizations have a unique opportunity to exceed Health Insurance Portability and Accountability Act (HIPAA) requirements due to the advanced personal identity record protections it affords. Companies conducting business in the global marketplace can also get a leg-up in meeting the EU’s General Data Protection Regulation (GDPR) designed to further informational privacy.
Look for skilled, automated managed detection and response rervices
Perhaps the greatest benefit organizations garner from AI and machine learning security automation is the ability to detect, respond and expel threat actors and malicious applications. Managed IT cybersecurity experts can help companies close the skills gap by integrating these and other advanced security strategies.
This feature was originally published on ISAGCA.
About The Author
John Funk is a Creative Consultant at SevenAtoms. A lifelong writer and storyteller, he has a passion for tech and cybersecurity. When he’s not found enjoying craft beer or playing Dungeons & Dragons, John can be often found spending time with his cats
Did you enjoy this great article?
Check out our free e-newsletters to read more great articles..
Subscribe